Medicine
Pathologists use AI to more accurately diagnose cancer. Data on different types of cancer are used to create a predictive model. For example, PathAI technology is used for this purpose.
Creation of medicines
Creating new drugs is a time consuming process because you need to do a lot of testing to find a useful formula. AI helps in this. Atomwise is one example of a technology that can detect new molecules. It is used to create new drugs for 27 diseases in collaboration with Harvard and Stanford universities and pharmaceutical companies.
Optimized patient data processing
The number of patients around the world is growing daily. Automated systems are required to process data on their diseases. AI allows medical institutions to optimize this treatment. For example, OLIVE is a platform for automating healthcare tasks.
Banking
Banks use machine learning to serve customers, predict risks, prevent risks and invest. For example, you can offer personalized offers based on the user’s financial behavior. Thus, if the client is looking for a home, he can make a special offer. Envestnet is a financial data collection and analysis company that provides financial management services.
Our Client History
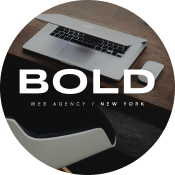
We are the top-rated web design company in North America. We will provide a quality update for your business, from redesigning sites of any complexity to website development from scratch.
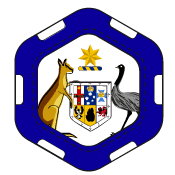
Try the list of the best online gambling sites made my TopAussieCasinos.com gambling experts team.
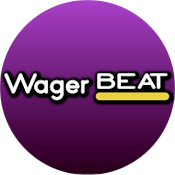
One of the best aussie casino brands – Wager Beat Casino – accepts not only usual fiat, but also complex cryptocurrencies developed using various technology sets. We helped with the integration of various wallets, blockchain monitors and transactional email notifications.
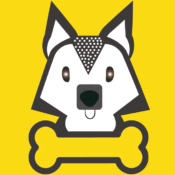
Exploring Vue.js might be mind blowing and you need to focus however my super active dog was distracting me all the time. I found the best CBD-infused dog treats to calm my pup and it perfectly worked!
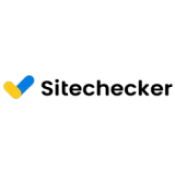
SEO checker by Sitechecker is the easiest On-Page SEO website checker program to help you improve your website and get more traffic from Google organic search.
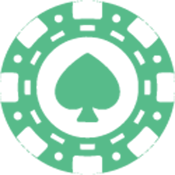
If you enjoy playing for fun or for real money, free slots are a great way to do that. Free online slots offer a variety of different games that you will never find in a land base casino.
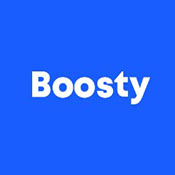
Boosty Labs is an outsourcing company specializing in fintech development.
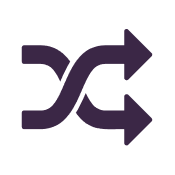
Service for renting individual proxies PROXY-STORE.COM will provide you with high-quality fast proxies at very competitive prices. Once applying our IP proxy-servers or any IPv4 sock proxy, you can no longer live without them.
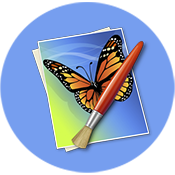
SoftOrbits Photo Retoucher is the Software to remove date from photo that can perfect your photos, making you want to frame them and hang on a wall.
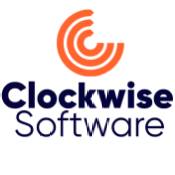
Clockwise Software is a dependable technology partner providing angular development services. We help businesses discover the bottlenecks in their working processes and eliminate them with software tailored to their specific needs.
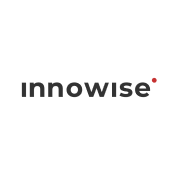
Innowise excels in mobile app development services, crafting innovative and user-friendly applications tailored to clients’ needs. With a skilled team, they ensure high-quality development, seamless integration, and ongoing support for business success in the mobile ecosystem.
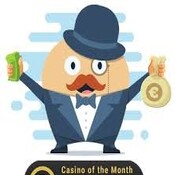
Wondering how to deal with boredom? Once you move a sign up at Mr Bet gambling establishment and use Mr bet login, you may enjoy a lot of positive points.
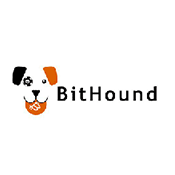
Are you looking for a reliable bitcoin casino? Read this 7bit casino review, written by BitHound.io team of experts in crypto & online gambling.
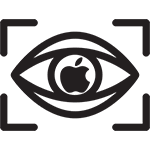
RetinaMacapps – knowledge base that provides easy workarounds for complex macOS problems and helps Apple users to choose only the best accessories for their Macbooks
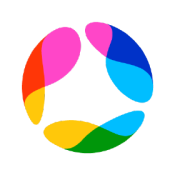
Avenga offers comprehensive business technology consulting services that help organizations ideate, validate, design, engineer, test, deploy, and maintain sophisticated technology solutions, accelerating digital transformation with advanced analytics and artificial intelligence.
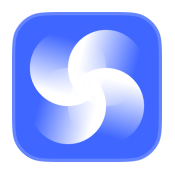
Fantastic image compressor was built using some of our insights in the field of photo size optimization.
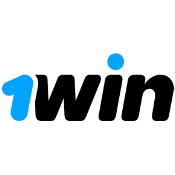
Discover exclusive rewards at 1win Brazil top online casino, with the bonus code 1win – 1wincombr. Visit 1win.com.br/bonus for special bonuses and elevate your gaming experience.